Satellite
Data
Elevate agriculture with remote sensing Precision data for optimal management

Agriculture background
The progression from Agriculture 1.0 to 4.0 signifies technological evolution in farming. Agriculture 1.0 relied on basic tools and human labor, while 2.0 introduced machinery. Agriculture 3.0 embraced data-driven practices, and 4.0, or “smart farming,” integrates AI, IoT, and big data for optimized, sustainable agriculture.

Agriculture 1.0
In the era of natural economies, smallholder communities predominantly depend on human and animal labor, with a primary focus on combating famine and ensuring food security.

Agriculture 2.0
Amid the commodity economy’s reign, agricultural commercialization leans heavily on machinery and equipment, striving for controllable production to meet demand and ensure stability in output.

Agriculture 3.0
In the capital economy era, financial agriculture thrives on internet connectivity and smart device utilization, prioritizing large-scale, tailor-made production to meet evolving market demands efficiently.
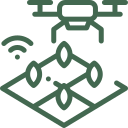
Agriculture 4.0
In the information economy era, digital agriculture leverages industrial integration to optimize resource allocation on a broad scale, aiming to enhance efficiency and sustainability in agricultural practices.
- International open source satellite
- Chinese civil satellites
- Commercial satellites
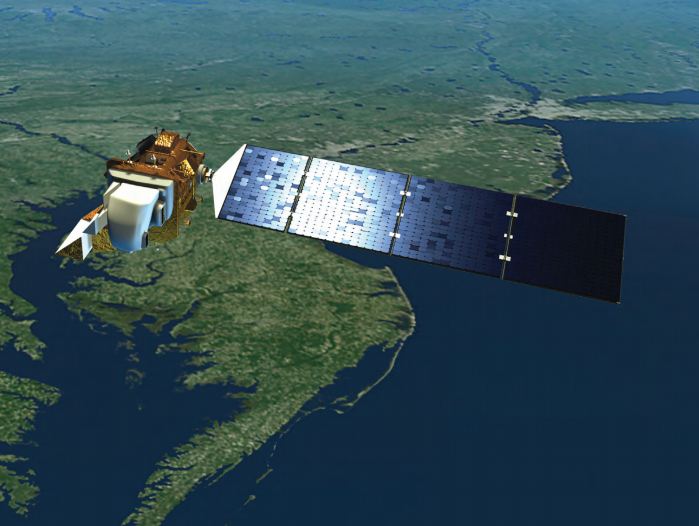
Sentinel
International Open Source Satellite
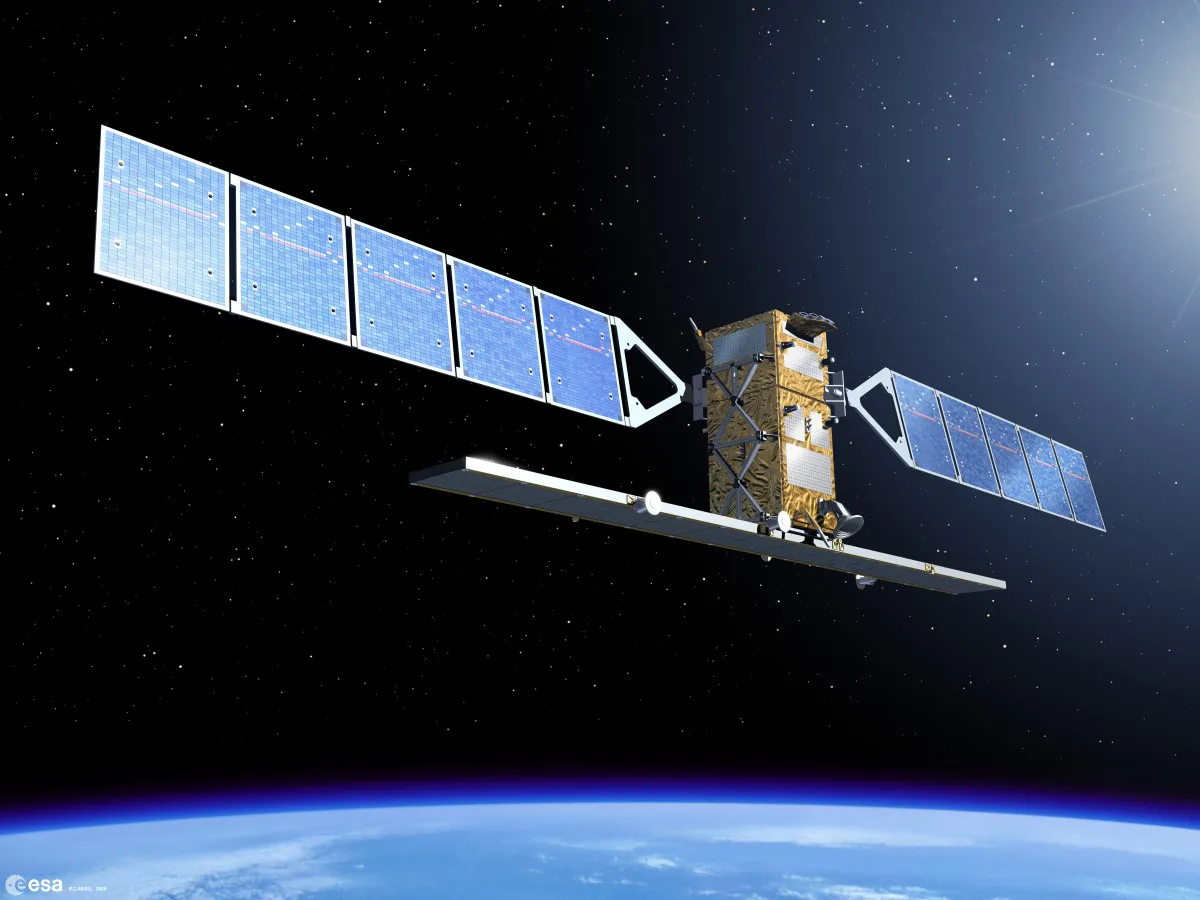
Landsat
International Open Source Satellite
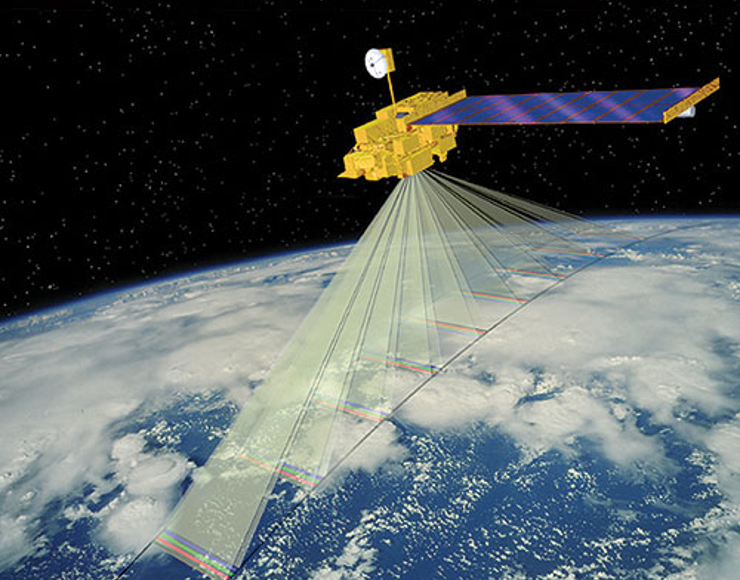
Terra (MODIS)
International Open Source Satellite
Application products and services for agriculture
A comprehensive array of data sources including RS satellite, meteorological, observational, and historical statistics converge to support agricultural operations. These encompass pre-tilage monitoring, planting surveillance, growth management, and harvest services. Derived application products and services span plot identification, quality assessment, planting area analysis, soil and growth monitoring, disease surveillance, and yield estimation.
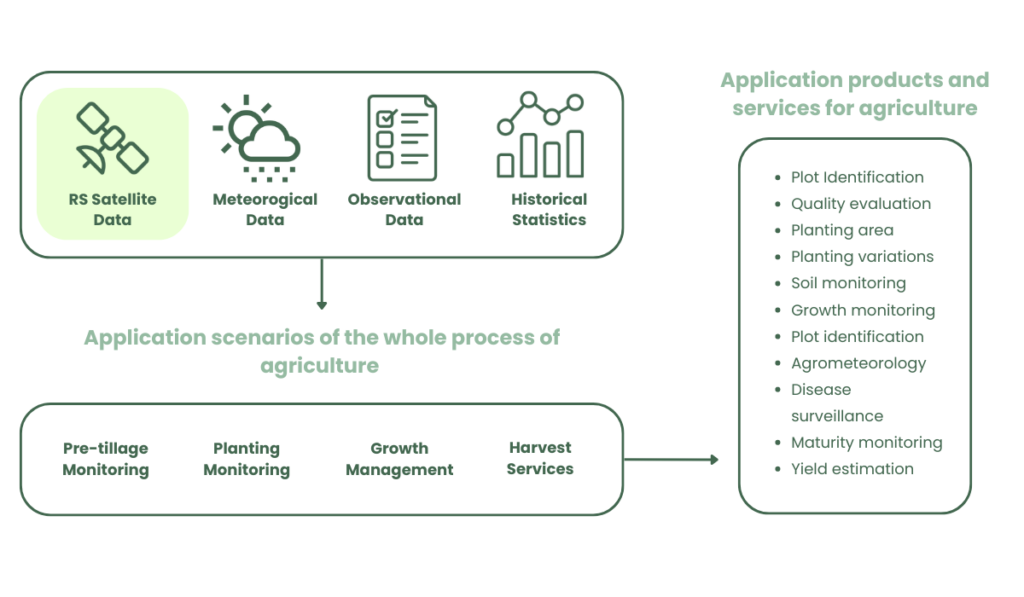

Application Products and services
Satellite remote sensing offers extensive support across agricultural production stages: plowing, planting, management, and harvesting. Its strengths—wide coverage, multi-band data, and geographical flexibility—enable diverse applications, providing crucial decision-making support for farmers throughout the agricultural cycle.
Pre-tillage monitoring
Recognizing cultivated land plots supports agricultural management, while evaluating cropland quality offers insights into soil health and productivity, aiding informed cultivation decisions.
Planting monitoring
Automatically identifying crops streamlines agricultural processes, while monitoring changes in planting patterns provides valuable data for adjusting cultivation strategies and optimizing yields.
Growth management
Monitoring crop growth, soil moisture, and fertility optimizes conditions. Disease identification prevents losses, and agrometeorological services provide weather insights for informed agricultural decisions.
Harvest services
Tracking maturity levels aids in optimal harvesting timing. Production estimation forecasts yields, crucial for planning and resource allocation, ensuring efficient agricultural operations and market responsiveness.

Siwei Preferential Remote Sensing Service Platform
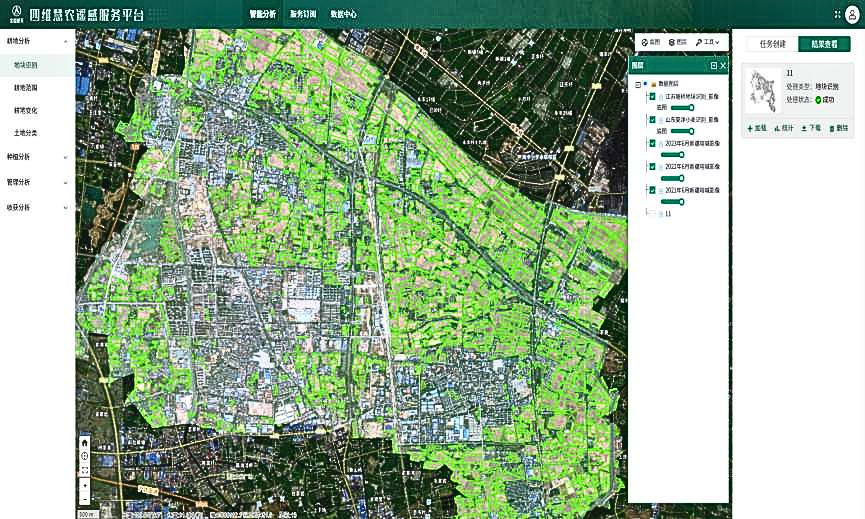
Intelligent Analytics
Utilizing online intelligent analysis, including the aforementioned methods, alongside self-developed AI algorithms enhances agricultural decision-making. Farmers can upload models for online analysis, enabling efficient utilization of advanced technology for improved productivity and resource management.
Data Centers
With access to 41 remote sensing satellites, including domestic and foreign ones, and control rights over 34 satellites, users can access images online and upload personal data for analysis.
Subscriptions
Offering subscription services for 28 products, including original images, result data, and specialized reports, the platform supports data downloads and API interface calls for seamless integration into users’ workflows.
What makes precise field boundaries important?

Pre-production
Pre-production activities, such as VRT, are vital for fungicide, herbicide, and pesticide companies, as well as plant breeding firms, necessitating precise field boundaries to optimize agricultural practices effectively.

Processing & storage
Grain traders, mills, and logistical networks rely on accurate field boundaries and real-time data on seeded acres during the growing season to forecast grain volume inventories effectively.

Wholesale & retail market
Grain buyers, food processors, and carbon crediting bodies depend on precise field boundaries and seeded acreage for carbon and sustainability mapping, emphasizing the importance of accurate agricultural data for environmental initiatives.

Autonomy & robotics
In-field autonomous agricultural machinery relies on highly precise boundaries for dependable operations. Traditional RTK boundaries are costly and lack scalability, highlighting the need for more accessible and scalable solutions.

Distribution & logistics
FMS providers aim to onboard and expand their user base efficiently. Manual digitization of field boundaries by farmers is prone to inaccuracy and time-consuming, underscoring the need for more streamlined solutions.

Crop insurance & subsidies
Crop insurance firms and national agencies often rely on manual field inspections for claims and subsidies, which proves both costly and time-consuming. Streamlining this process with digital solutions can greatly enhance efficiency and reduce overheads.
Deep Resolution Imagery
Deep Resolution Imagery Is essential to modern agriculture
Land Use Planning
Analyzing soil types, topography, and water availability guides crop allocation, cultivation decisions, and land use optimization, enhancing agricultural productivity and sustainability.
Pest and Disease Management
Early pest and disease detection empowers farmers to intervene promptly with targeted measures like pesticide application, minimizing crop losses and promoting healthier yields.
Precision Farming
Detailed crop variability information enables precision agriculture techniques like variable rate input application, optimizing resource usage and saving costs for farmers.
Yield Prediction and Forecasting
When paired with machine learning, it predicts crop yields, aiding in marketing decisions and optimizing supply chain management, empowering farmers with foresight.
Crop Monitoring
Monitoring crop health and growth, analyzing imagery data aids in stress or disease identification, optimizing irrigation and fertilization, and maximizing productivity through informed decision-making.
Different crops
Not just for main crops like wheat, barley, rye, and oats, but also for more diverse crop-specific data, which will improve overall accuracy.
Crop Training Data
Over 100,000 field level crop seeded data points collected over a 5-year period provide the validated and trustworthy ground truth data that the model is based on.
Classification of crops
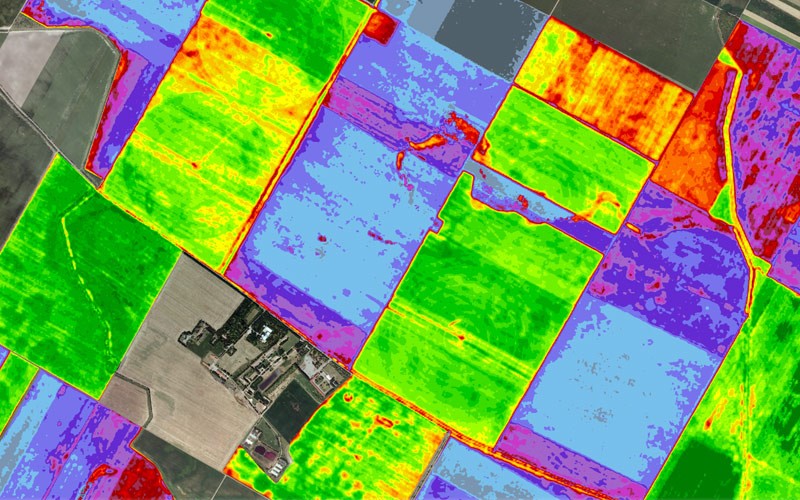
High Accuracy
The improved field delineation model accuracy and the merging of S2 and S1 data achieved 92%.
Project Based
Crop Classification in the form of projects with varying degrees of difficulty and specificity levels.